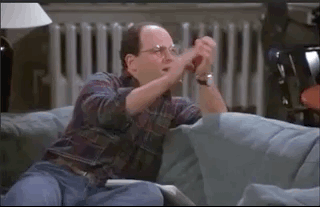
Experience a transformation in your forecast predictability with Funnelcast, a statistical model solution that empowers your teams to improve their sales practice. But that's not all – Funnelcast excels in enhancing forecast accuracy. Dive deep into customer insights, enabling your team to make real-time, data-driven decisions confidently. Elevate your win rates and forecast accuracy with Funnelcast, the solution that brings you the power of predictive analytics.
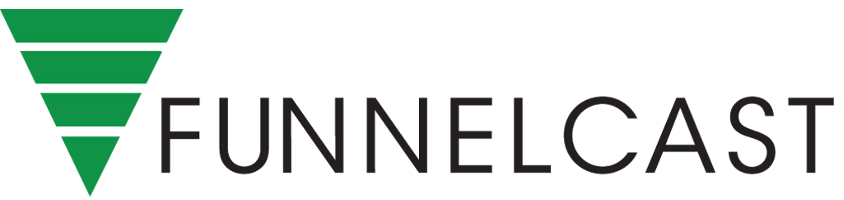
According to a study in 2021 by the RAIN Group Center for Sales Research, the overall average conversion rate (across various sales industries) is 47%. However, other research has found that the SaaS win rate benchmark is lower, closer to 22%. The team surveyed 472 sellers and sales executives in organizations with at least 10 sales reps to 5,000+.
HubSpot's survey on close rates of over 1,000 sales professionals revealed that 53% of respondents saw their close rates remain relatively stagnant from 2021 to 2022, while 35% reported an increase and 12% reported a decrease. The survey highlighted the importance of lead quality, marketing, sales alignment, and customer relationship management in influencing close rates. Notably, 25.9% of respondents saw improved lead quality as a significant benefit of well-aligned sales and marketing departments. Furthermore, 56.3% of salespeople generated leads through customer referrals, considered the highest-quality leads.
Okay, the win rate is not the same as the close rate, but it sets the stage here well before we get into the deliberation about improving the former. But first, the metric definition.
The formula is straightforward and probably doesn't require a lot of deliberation:
Win Rate% = (Number of deals you win / Number of deals you pursue) x 100%
For example, your business pursued 50 deals last month and won 10. Calculating the win rate is: (10/50) x 100% = 20%
Your sales win rate for the business in that month is 20%.
The real fun starts when you need to provide narratives, including the "why" behind such disappointing results. There are several reasons, although they only apply to some products or services companies provide. There is always it's flavor. However, if you take top-performing companies on that metric, you should be around 60%. Even stock traders operate around a 50% success rate, making much money on trades they win versus those they lose. Ultimately, you are in the business of predictability, not a guessing game. Yes, things can change, and you can alter that number based on what you don't know, but in the end, it's zero or one at the end of the contract or period you are measured against.
So, how do you improve your 20% or less by trying to bridge this to the best in class? First, let's explain why we need to improve that ratio. One reason is that the SaaS sales cycle is often complex and lengthy, with multiple decision-makers involved, many teams influencing the opportunity, touching it, etc. This can make identifying the key factors affecting a customer's buying decision challenging. The SaaS industry constantly evolves, with new products and features released continuously. There is a constant pull or push from your competitors as well. It can make it challenging to keep up with the latest trends and accurately assess customer needs.
Despite the challenges, there are several things that companies can do to improve their win rate prediction accuracy. One crucial step is to collect and analyze historical sales data. This data can be used to identify patterns that can help to predict future sales outcomes. It can help you create a customer profile you should be approaching. It can help you define your ICP, buying persona, and anything you need to know about your product or offering resonating on the market. Nothing here is in absolute terms, and let me remind you that we focus on improvement, not perfection. Even a couple of percentage points improvement can make you a lot of money. Before we get into the "the past is not always predicting the future" convo, I want to admit that we must keep that in mind. Yes, adjustments are inevitable, as we underlined above about constantly changing conditions. However, this should make us think we can only drift with something we continuously monitor and adjust. Yes, some things in our planning are static and need to be more flexible, but we still have to keep the whole idea. That's the side note that I need to make for the sake of the quality of the debate here.
Additionally, companies can use sales forecasting tools to automate the process of win rate prediction. The mysterious PLG occurred somewhere in these conversations. The FCSTing tools can consider factors such as the customer's industry, size, and budget to generate more accurate predictions. The elephant in the room is your rep's behavior and the source of the deal. GTM often includes these sources but needs to analyze the behaviors of those driving and responding to them more. Let's take partnership/ channel sales as an example; from the output perspective, it would be easier to see what will close if analyzing these behaviors at the aggregate level. A similar would be with direct sales and marketing. That's where the statistical modeling can help. Let's not be scared; this doesn't have to be complicated or revolutionize what you do. The underlying data must be in good shape to increase your prediction accuracy. However, even if that's not the case, you will get a good understanding of where you are going. The absolute no-go is when you have no data, but I know this is obvious to our readers.
This pivotal measure of sales and marketing success is facing one fundamental challenge within the leaders in most companies in pursuit of fast-scaling organizations. Reflections of their committed numbers often refer to something beyond the best-case scenario that numbers tell you. Everything is hinged on people's aspirations instead of realistic expectations driving the market and your organization's abilities (including product). For the same reason, many companies stumble in setting realistic expectations around this crucial ratio, often leading to skewed performance evaluations and misguided strategies.
I want to focus on the typical issues around that practice:
Sales process simplification (or rather oversimplification): One of the significant challenges is the tendency to oversimplify the sales cycle. Sales processes in the SaaS sector can be intricate, involving multiple stages and stakeholders. When companies need to recognize this complexity, especially the variability in the length and nature of the sales cycle for different customer segments, they set win rate expectations that do not reflect the real challenges sales teams face. There is plenty to unpack here, but for now, we get it.
Neglecting the customer base: Another critical oversight needs to consider the diversity of the customer base. SaaS products often cater to a wide range of customers, from SMBs to large enterprises, each with unique needs and decision-making processes. Ignoring these differences can lead to a one-size-fits-all approach in sales strategies, which is rarely effective and leads to unrealistic win rate targets. Well, you've seen me many times saying this. Everything starts with your product. Here is the same. It can fit a big company or industry, not necessarily a small or another sector. Sounds obvious? Then why is everyone saying we are moving to enterprise sales without asking if there is a product fit for that?
Reliance on Outdated Data: Many SaaS companies rely heavily on historical sales data to forecast future performance. However, this approach can only be misleading if the data is updated or needs to consider recent market shifts and evolving customer needs. The rapid pace of change in the SaaS industry means that what worked in the past may not indicate future success. Many companies do not restate their numbers after significant changes occur. What you sold two years ago might not be relevant to the same customer base if you had major changes in your product design or release, acquisition of new products, or consultative sales process versus point solution.
Alignment between functions (rather a lack of alignment): Effective sales strategies require a cohesive approach that involves sales, marketing, product development, and customer support teams. A lack of alignment between these departments can lead to inconsistent messaging, misaligned goals, and ineffective sales tactics, negatively impacting the win rate. For instance, if the marketing team is not aligned with the sales team's approach and target audience, the leads generated may not be of high quality, thus affecting the win rate. Guess what? That will impact your pipeline generation, velocity, and, ultimately, your win rate. These things go in volumes of lost money over 6 or 12 months.
Okay, we know the problems; let's spend some time on the methods that can get you out of this madness:
Companies utilizing suitable statistical models for their sales team's pipeline tend to see markedly improved performance. These models provide a more accurate and realistic picture of the sales landscape, enabling better forecasting and resource allocation. The difference is often quantifiable in increased conversion rates, more efficient sales cycles, and a higher overall win rate. Here are some extras you can focus on:
Historical sales data analysis: it takes diving deep into past sales records to identify patterns, trends, and anomalies. Analyzing historical data helps understand which strategies worked, didn’t, and why. This analysis should go beyond just looking at numbers and include factors like sales cycle duration, response to different sales tactics, and customer retention rates. You need to have a wall with these metrics that are constantly changing and monitor deviations against your past and current performance.
Predictive analytics: utilizing statistical models and machine learning algorithms to forecast future sales outcomes based on historical data. This method can reveal insights that are not immediately apparent from a simple review of past sales data, such as subtle trends or emerging market shifts. You must remember that this is flawed, with one thing you won't get from it. If you pivot into something else, these models need a feed from another source. Products on the market forget that although that's not easy to solve. I can't blame them.
Lead Scoring: Lead scoring involves assigning a value to each lead based on their changes to convert into a customer. Factors like demographic information, engagement level, and specific interactions with the company’s content or products are used to score leads. This system helps sales teams prioritize their efforts on leads more likely to convert, thus potentially improving win rates. Narrowing down helps your win rate focus on the correct targets you want to acquire. You need to pre-select the proper fit entities in the first place.
Sales Stages: Regularly reviewing and optimizing the sales funnel stages is crucial. This might involve redefining the criteria for moving a lead from one stage to another or introducing new stages to capture the nuances of the customer journey better. A well-defined sales funnel ensures that leads are nurtured effectively at each stage, increasing the chances of conversion. Now, the most crucial part is the process that reflects the pattern of the decisions made by your prospects and customers. You need to reflect on the process of their thinking, not how you want sales to be. You can't adjust this, but having a check-in every 6 months and adjusting is not a bad practice. Just ensure you have enough data samples to call the change. If not, wait for the entire 12-month cycle.
Market Analysis: Staying updated on market trends and competitor activities is critical. Regular market analysis can provide insights into emerging customer needs, competitive strategies, and market opportunities. The market analysis information can be used to adjust sales strategies quickly. Again, you can only change your strategy occasionally, but you can learn from the changes, create a hypothesis, and test it. You should consider implementing that in your strategy if there is enough evidence.
Customer Feedback: Direct input from customers can provide invaluable insights into their needs, preferences, and pain points. This feedback should be integrated into sales strategies to remain relevant and practical. Customer feedback can also help refine product offerings and customer service approaches, improving win rates.
Conclusion: There is no silver bullet to the predictive analysis around the win rate. However, there is a set of actions we can take to get us closer. One of them is creating a set of metrics that are critical milestones in converting prospects from early-stage to late-stage opportunities. Other metrics must be closely monitored to maximize the effectiveness of win rate predictions.
These include the sales cycle length, average deal size, lead-to-opportunity ratio, customer acquisition cost, conversion between the stages, etc. Keeping an eye on these metrics, alongside a robust win rate analysis, equips your teams with a toolkit for navigating the competitive and ever-evolving landscape of SaaS sales. Let's look at these metrics and how to think about them in the win rate context:
Length of the Sales Cycle: It is crucial to monitor how long it takes to close a deal. A shorter sales cycle indicates a more efficient sales process and a product-market fit. In comparison, a longer cycle might suggest potential bottlenecks in the sales process or the need for better qualification of leads. Understanding the average sales cycle length helps forecast sales timelines and manage resources more effectively. Your consultative selling can't have a 3-month sales cycle, but point-solution can have even 30 days. Assuming you're out of the range for your sales methodology linked to your product, you should work harder to get that under control to improve your win rate. Although that won't happen automatically, it can still be an essential improvement.
Average Deal Size: This metric provides insights into each customer's value to the business. Tracking the average deal size over time can reveal trends in customer spending behavior and help adjust sales strategies to target higher-value deals or understand the impact of pricing strategies on sales outcomes. Again, if your win rate looks terrible, you must see the lost and won reasons and determine if those impact the average deal size.
Lead-to-Opportunity Ratio: This ratio measures the effectiveness of the lead generation and qualification process. A high ratio indicates that many leads are qualified as opportunities, suggesting effective lead generation and initial sales processes. However, if the ratio is low, it might point to lead quality issues or the sales process's initial stages.
Customer Acquisition Cost (CAC): CAC is a critical measure of acquiring a new customer, including marketing and sales expenses. Keeping track of CAC about the lifetime value of a customer (LTV) is essential for ensuring the company's long-term financial health. A lower CAC relative to LTV indicates a more sustainable and profitable business model. This metric may not be evident to most of us, but to me, it is. Resource allocation is an essential factor behind all your adjustments. Let's say you observed an uptick in the partner-sourced deals for the last 6 or 12-month period with a shorter selling cycle than direct sales or marketing-sourced deals. It is when your win rate can improve, allocating more resources to the right team.
Comments